
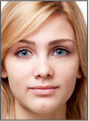
Segmentation models predict pixel-level masks for green-screen-like effects or photo composites. If the AR effect you’d like to implement doesn’t need to be connected directly to the component being classified (in this case, the range of desserts themselves), then a classification model should work well.

Notice that the confetti effect above isn’t targeted or localized within the camera scene-instead, the prediction triggers an effect that isn’t directly attached to the object being recognized. For example, you might have a classification model that can see and recognize a variety of different dessert food items, as shown in the gif below: Often, that prediction is mapped to a text label that can either be shown to end users or trigger a global AR effect. In this blog post, I want to try to demystify at least one part of this equation-what’s actually creatively possible with SnapML in Lens Creation.Īlso commonly referred to as image labeling and image recognition, image classification is a computer vision task that simply predicts what is seen within an image or video frame.
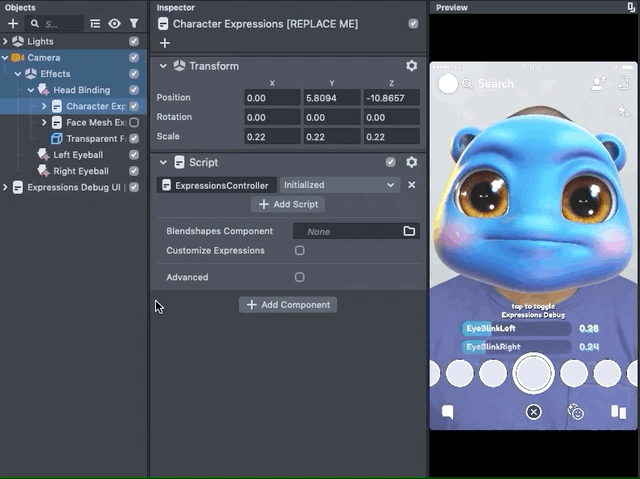
This new set of capabilities quite literally extends what Creators can do with Lens Studio, allowing them to bring in completely custom ML models.īut the truth is, machine learning isn’t always easy to understand conceptually, much less actually implement in Lenses. With SnapML, though, Snapchat has opened up this expansive capability to its community of Lens Creators, allowing them to implement custom machine learning models in their Lenses. Machine learning unlocks a wide range of possibilities when it comes to AR creation, and the two technologies can be quite complementary-nobody in the industry has proved that to be true more than Snapchat, whose immersive AR Lenses work so well in large part because of the complex ML models running underneath them (things like face tracking, background segmentation, and more). Without further ado, let’s hear it from Austin! 👇
#Lens studio face expressions software
Austin will tell you all about possible use cases for utilizing their software to create many types of ML-enabled, powerful AR experiences. As promised, we come to you with the second part of our SnapML x Fritz AI article, this time with a guest post from Austin from the aforementioned Fritz AI.
